Data Annotation for Financial AI: How U.S. Banks Are Leveraging High-Quality Data 15 May 2025
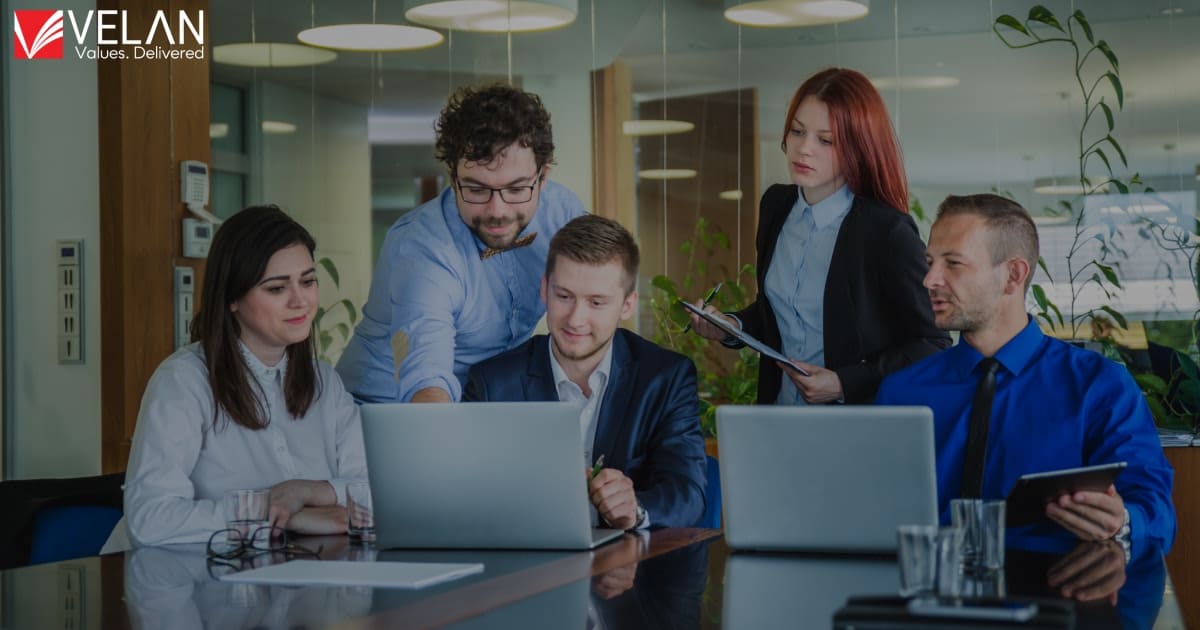
AI is transforming sectors all over the globe, and arguably the most impacted industry is that of finance, considering the multitude of applications from fraud management to credit analysis. One such “quiet revolution” that changes the financial landscape is annotated data for financial AI algorithms. The banking industry in the US heavily relies on credit and risk-assessing algorithms, and their outcome is highly dependent on the training data. This illustrates the importance of data for financial AI.
The Growing Role of AI in the U.S. Banking Industry
Major U.S. banks, including JPMorgan Chase and Wells Fargo, are progressively utilising AI solutions to optimise operations, mitigate risk, and provide more personalised services to their customers. Currently, financial AI works for several applications, such as:
- Payment Defaults: AI systems studying payment behaviour and history tend to analyse present defaults.
- Fraud Detection: Suspicious transactions can be detected automatically by real-time systems preemptively before being finalised.
AI systems are currently not equipped to handle raw, unordered, unprocessed information. Labelled datasets that are meticulously structured and well-kept are essential to these systems working efficiently.
Data annotation works in altering the extensive amounts of financial information in a format that is comprehensible for machine learning algorithms so that they can be trained efficiently. Robo-advisors can manage user equity funds with ease through algorithms based on machine-learning investment strategies along with modern programming languages. Accessing their investment is simple for users via a website or app.
Mobile applications have made investing easier today, enabling even those unfamiliar with technology to operate them with a device in their hands. The use of these devices aids domain novices in making educated and beneficial decisions. These technological luxuries are projected to improve financial literacy levels worldwide.
Why Data Annotation Matters for Financial AI
The labelling of various categories of datasets, such as transactions, loan applications, chat conversations, or legal documents, is what comprises data annotation in finance, followed by processes executed in classification spanning processes of consolidating documents to sorting reliable transactions and automating the repetitive tasks in finance.
- For example, within fraud detection, annotators mark up historical transactions as “legitimate” or “suspicious.”
- These labels help train machine learning models to detect certain patterns of fraud, like multiple failed login attempts or large purchases made out of context.
- The AI gets better at detecting suspicious activity that is new and thus not previously seen over time.
- Without accurate annotation, the AI could learn incorrect distinguishing features.
- This can lead to being too permissive, where fraud gets overlooked (which actually is fraudulent), or overly aggressive, where legitimate transactions are questioned.
- Weak models can lead to irrational costs, erosion of reputation, and fines imposed by authorities.
As previously mentioned, high-quality model training requires relevant and accurate data supervision or annotation. Foundation models of many financial AI systems need clean data to produce reliable results.
The Employment of AI Annotations in US Banking
Leading banks now seem to integrate services with tailored data annotation companies or build in-house staff teams for comprehensive financial data annotation. The impact of data annotation for banking AI on model performance is reflected in this pattern.
It is clear from various case scenarios how U.S. banks apply data annotation to empower AI technologies:
- Risk evaluation: Data is labelled so that AI models can accurately predict risk using historical lending data.
- Automation of Customer Services: Chatbots are trained to converse with people accurately using labelled chat records.
- Fraud detection: Real-time fraud detection systems can rapidly detect anomalies due to the high-quality labelling of past fraudulent activities.
Benefits of High-Quality Data Annotation for Financial AI in the U.S.
In the U.S., the financial AI’s benefits that come from high-quality data annotation are countless.
For the case of high-quality data annotation, the financial AI’s pros in the U.S. are
Qualitative Improvement
AI operates more and more with greater precision when it comes to labeled datasets, and thus models trained on clean data perform at their best.
Labeling Compliance
Regulated compliance for banks is made easy when data ensures AI’s decisions are transparent through annotated data.
Justification Of Data Annotation’s Impact on Crafting Bank AI Models
AI can “learn” from financial data in a meaningful manner through data annotation. Consider it analogous to instructing a child: by providing them with examples of objects (such as pears and oranges) and accurately labelling them, they will eventually develop the ability to distinguish between them. AI operates in the same manner.
- AI models can recognize that banks use annotated data.
- Stock market patterns,
- Typical indicators of fraudulent transactions include
- Behaviours that are associated with the success or failure of loan repayment,
- Customer satisfaction or dissatisfaction trends.
For instance, to forecast the stock market, a bank must train the neural network with historical market data. The data must be accurately designated, for example, by tagging events that impacted the market (e.g., economic reports, company earnings, global crises). The artificial intelligence can learn to forecast more precisely as the more exact and detailed the annotation is.
In the same vein, NLP models that analyse customer feedback necessitate data that is accurately tagged. For instance, the phrase “I am satisfied with the service” could be classified as a positive sentiment. The negative sentiment “The loan process took too long” may be associated with the processing time. The AI’s performance is directly influenced by the quality of the annotation in both scenarios.
The Importance of Data Labelling in Financial AI and Machine Learning
Data labeling is not merely a back-end task in financial AI and machine learning; it is a fundamental strategic requirement. This is because financial institutions look after delicate, complex, and risky datasets. Poor interpretation can lead to suboptimal choices.
- Lapsed compliance matters.
- Inaccurate customer risk profiles.
The following cases show the need for data labelling.
- AI needs to retrieve some specific entities from the financial documents, like the name of the account holder, the account number, the interest rate, and the payment date. The elements are automatically recognized by the model with the help of labelling.
- Labelling in customer service files assists AI in understanding the context and attitude (positive, neutral, or negative).
- A label has to be attached to data sets if a model intends to change from basic pattern recognition to useful, meaningful insight generation.
High-Quality Data Annotation for Building Reliable Financial AI Models
The finance industry never compromises on precision and dependability. Take, for example, a financial AI model. If it predicts loan applicants who are of good standing and need financing as undeserving of such services or misclassifies transactions where payment fraud is attempted as innocent, massive losses, non-compliance with policies, and damage to trust follow.
To prevent this, we use prioritized, top-notch annotation services, which apply labels with such precision as to produce accurate results (no errors or misclassifications). Why are top-notch annotation services used, which are labelled with such precision as to produce
- Accurate (no errors or misclassifications),
- Consistent (applies the same criteria in the different datasets),
- Context-aware (understands the nuances of financial language and transactions)
To achieve such a high quality, several banks are adopting a hybrid annotation strategy:
Human annotators: These are experts in the domain or human teams who understand the financial data context, such as legal language, types of transactions, and regulatory vocabulary. They are especially useful in complex or delicate cases, as they ensure accuracy.
- Automated solutions: These tools use AI to accelerate or assist in the annotation. For example, algorithms can ultimately pre-annotate data, which humans can then review and correct.
- In this human-in-the-loop system, large amounts of data can be efficiently maintained by the institutions (scalability), and the accuracy of the data can be ensured.
Even a small mistake in a high-risk environment like finance can lead to dire consequences. This is why reliable financial AI models, safe to be introduced into actual banking processes, are made possible only by means of good-quality data annotation that can be realized by bringing together AI efficiency and human intuition.
Conclusion
Although financial AI is reconfiguring the financial landscape, U.S. banks using AI will need to focus on curating high-quality data for financial AI. It is not just more data; it is better data. The road to safer, smarter banking is made of well-labeled information, from annotated financial data to well-calibrated models.
By recognizing the importance of data annotation in predictive banking models, banks can leverage the power of machine learning to make better decisions, gain a competitive edge, and ultimately deliver more to their customers.